AI Readiness Assessment
Accelerate ROI with prebuilt, compliance-first AI Copilots tailored for your industry, built on Microsoft’s trusted ecosystem powered by CodeSizzler.
Identify Capability Gaps
Mitigate Implementation Risks
Optimize Resource Allocation
Current-State Maturity Audit
Deploy Copilot Agents that align with your compliance needs and business objectives—zero compromises.
- AI Infrastructure Health Check: Validate compute, storage, and cloud resources for LLM/Agentic workloads (e.g., GPU availability, API latency).
- Data Ecosystem Viability: Audit data pipelines, quality, labeling, and governance for AI training/inference compliance.
- Talent & Skills Inventory: Map gaps in critical roles (LLM engineers, AI ethicists, autonomous systems architects).
- Ethical AI Governance Review: Assess existing frameworks for bias detection, explainability, and human oversight.
- Process Automation Mapping: Identify workflows ripe for Gen AI augmentation (e.g., document processing, chatbots).
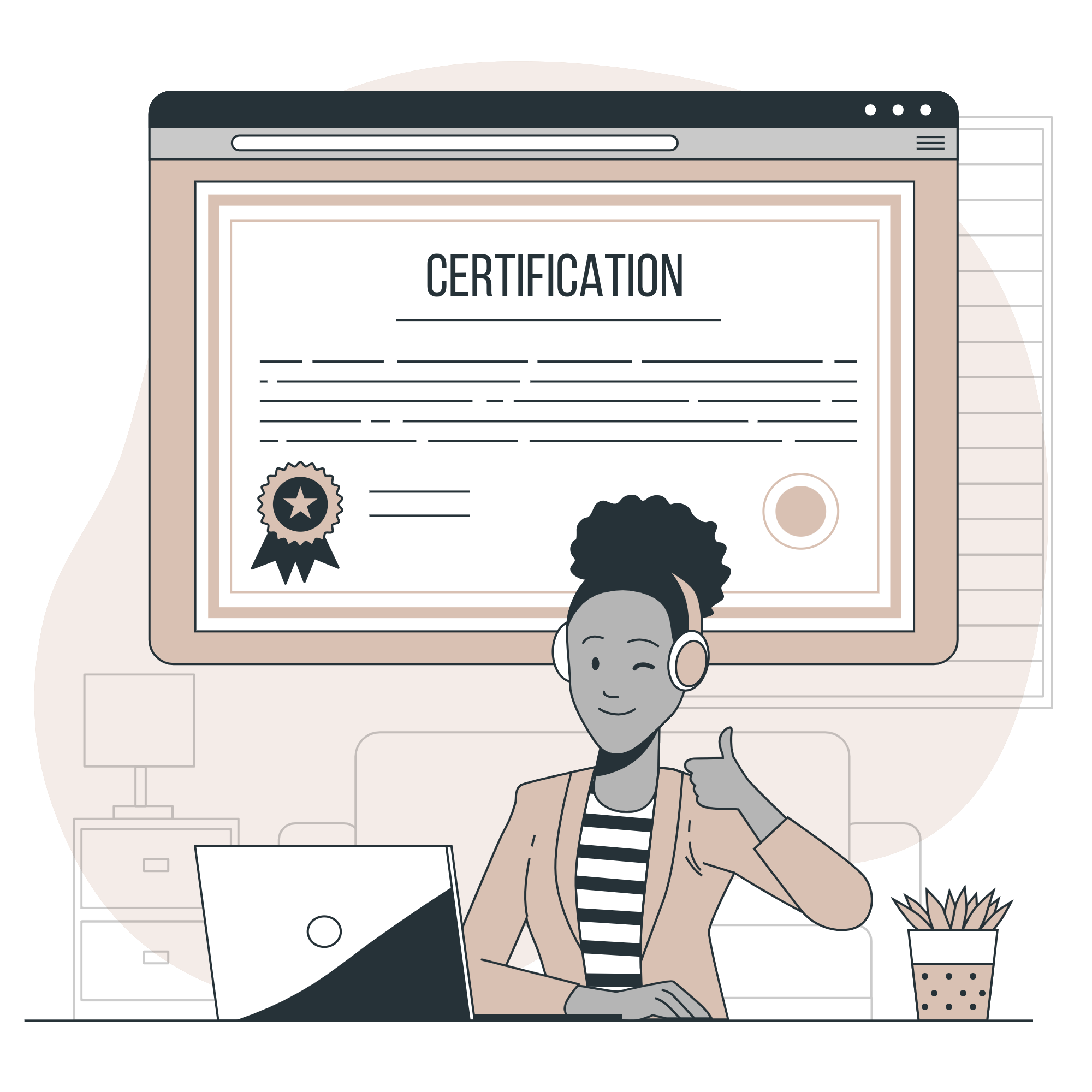
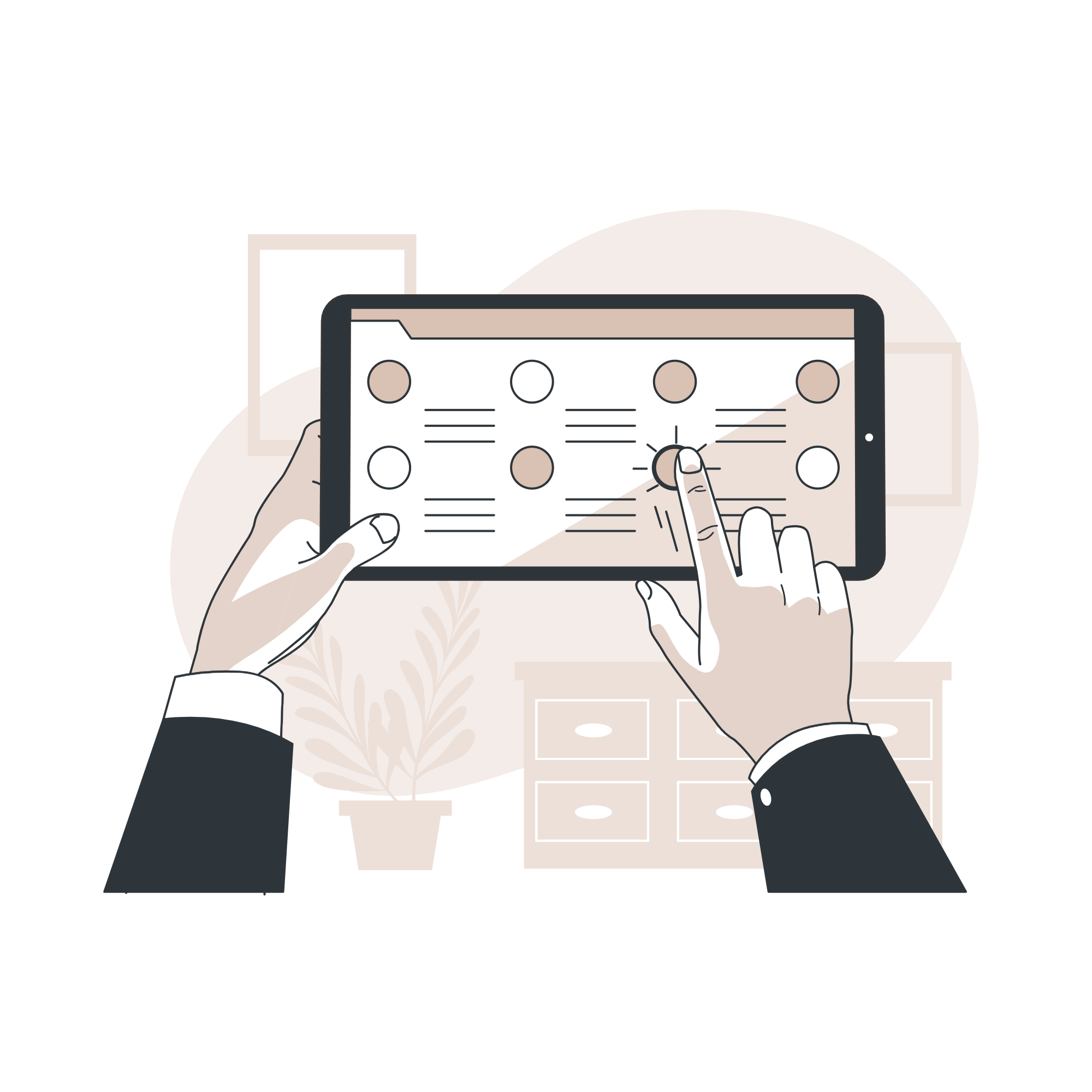
Be there before your competition
Strategic Use Case Prioritization
- High-Impact Scenario Identification: Rank use cases (e.g., AI copilots, predictive analytics) by ROI, feasibility, and risk.
- Regulatory Readiness Scoring: Flag use cases requiring compliance with GDPR, AI Act, or industry-specific mandates.
- ROI Benchmarking: Compare build-vs-buy options (e.g., OpenAI APIs vs. custom LLMs) for cost and scalability.
- Agentic AI Simulation: Test autonomous agent workflows (e.g., supply chain bots, IoT-driven decisions) in sandbox environments.
- Competitive Gap Analysis: Evaluate peer benchmarks to avoid lagging in AI-driven innovation.
Technical Architecture & Scalability
- Gen AI Toolchain Compatibility: Audit integration of vector databases, LLMOps tools, and existing ERP/CRM systems.
- Latency & Real-Time Processing: Stress-test infrastructure for Agentic AI needs (e.g., sub-100ms response times).
- Multi-Cloud Orchestration: Design hybrid architectures for seamless scaling of AI workloads across regions.
- Security Protocols: Review encryption, access controls, and data anonymization for AI model safety.
- Edge/IoT Integration: Validate infrastructure for Agentic AI deployments in IoT, manufacturing, or field operations.
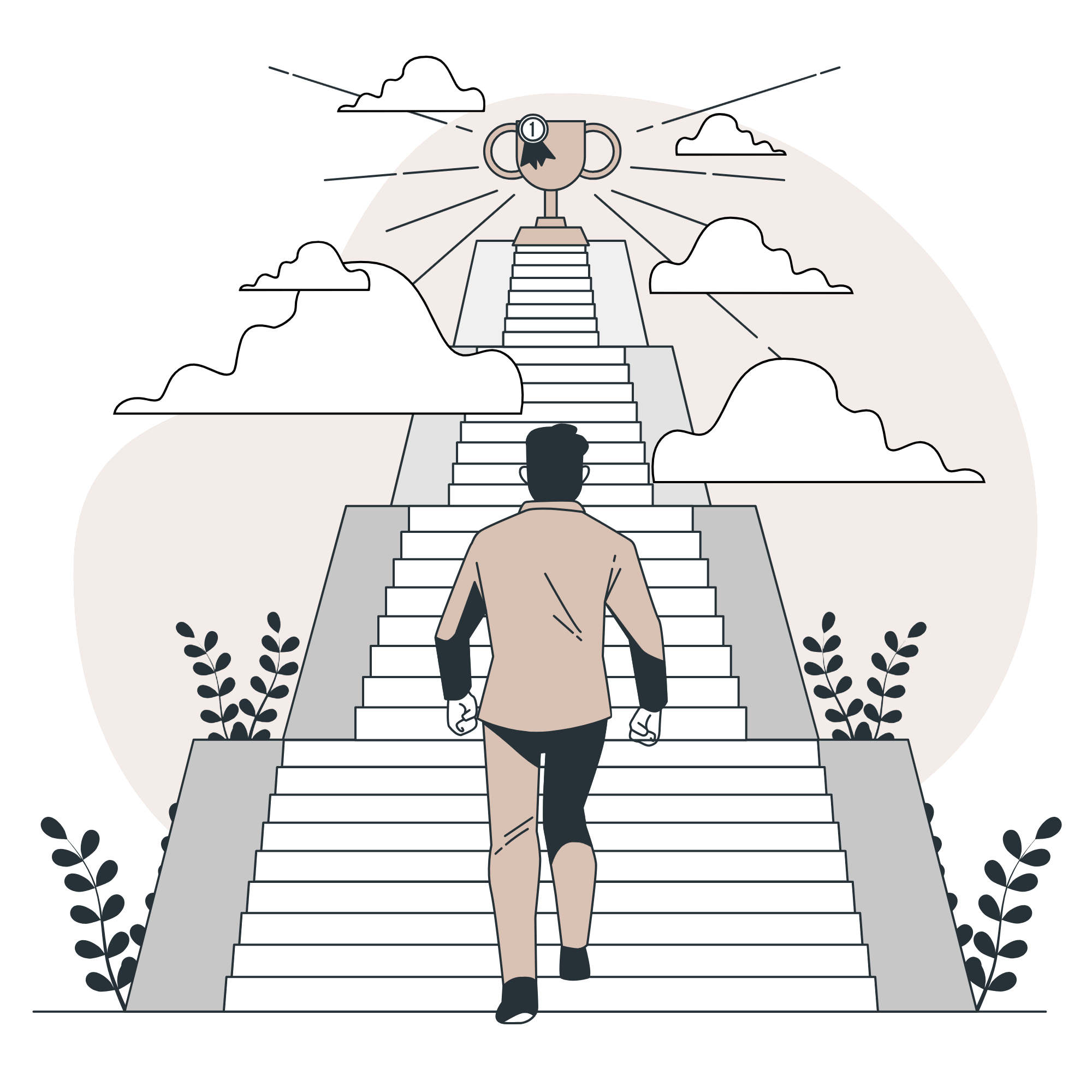
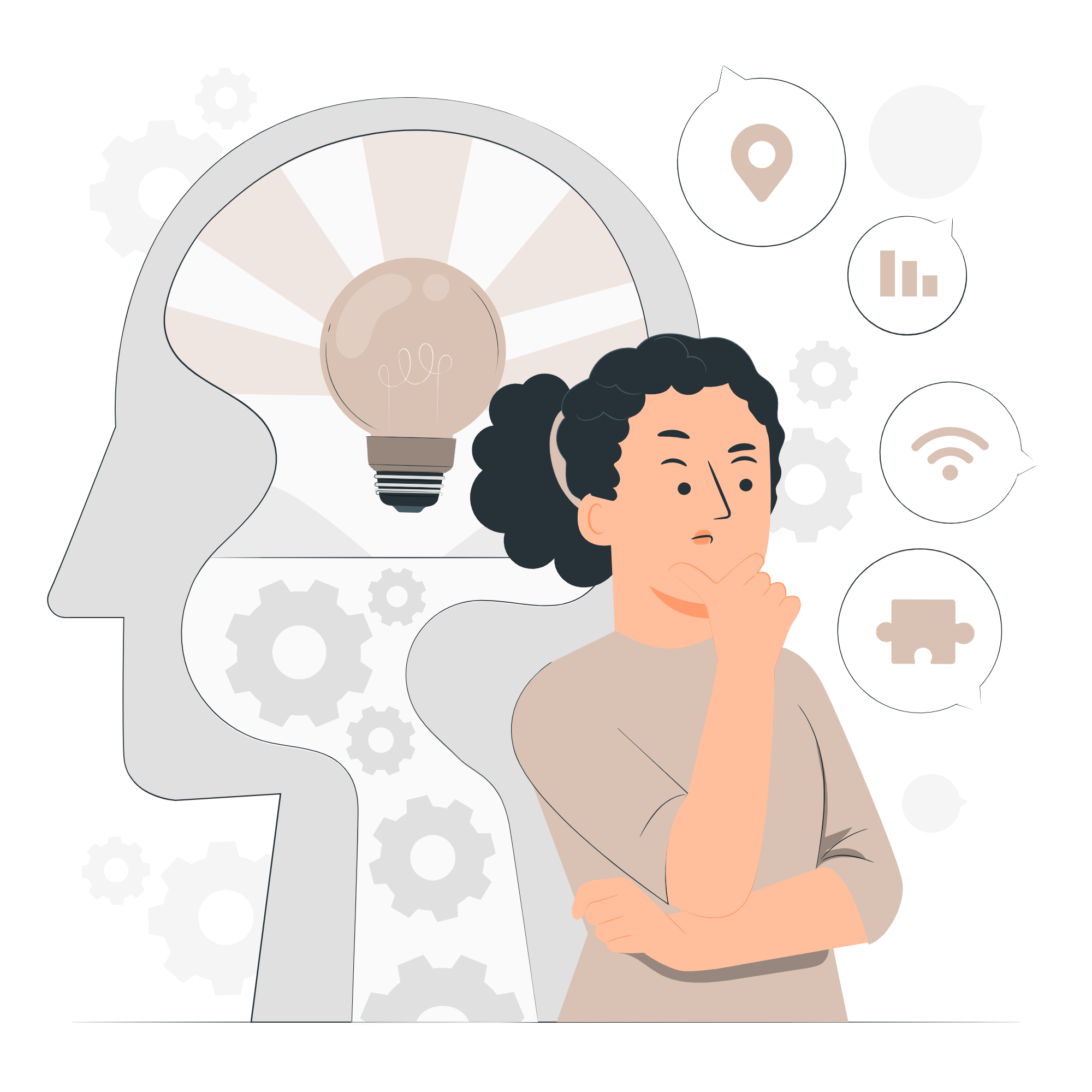
Be there before your competition
Risk Mitigation & Compliance Framework
- Bias & Hallucination Testing: Implement red-teaming for LLMs and autonomous agents to minimize harmful outputs.
- Explainability Requirements: Define thresholds for model transparency in critical workflows (e.g., finance, healthcare).
- Autonomy Liability Framework: Establish accountability chains for Agentic AI decisions (human-in-the-loop vs. full autonomy).
- Incident Response Playbook: Draft protocols for AI failures (e.g., misinformation leaks, agentic workflow errors).
- Regional Compliance Alignment: Adapt frameworks to EU AI Act, U.S. Executive Orders, and APAC regulations.
Implementation Roadmap & Talent Strategy
- Phased Rollout Plan: Define milestones (PoC → Pilot → Scale) with KPIs and budget allocation.
- Pilot Design: Build a 12-week sandbox for testing high-priority use cases with measurable outcomes.
- Change Management Blueprint: Address employee/customer adoption barriers with training and communication plans.
- Upskilling Partnerships: Curate programs for prompt engineering, AI ethics, and autonomous system monitoring.
- Vendor Evaluation Matrix: Score third-party AI tools (e.g., Microsoft Copilot Studio, AWS Bedrock) for fit and lock-in risks.
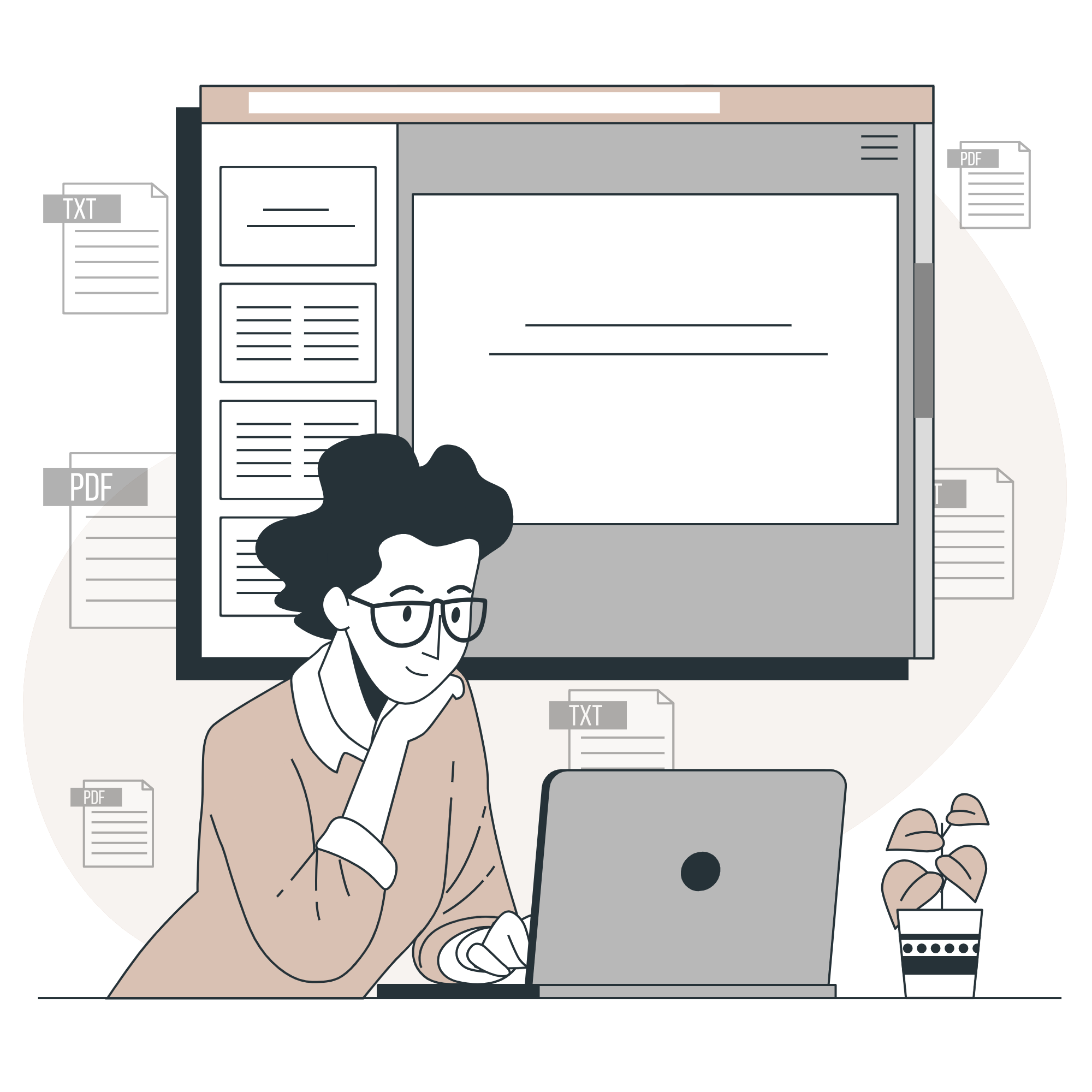